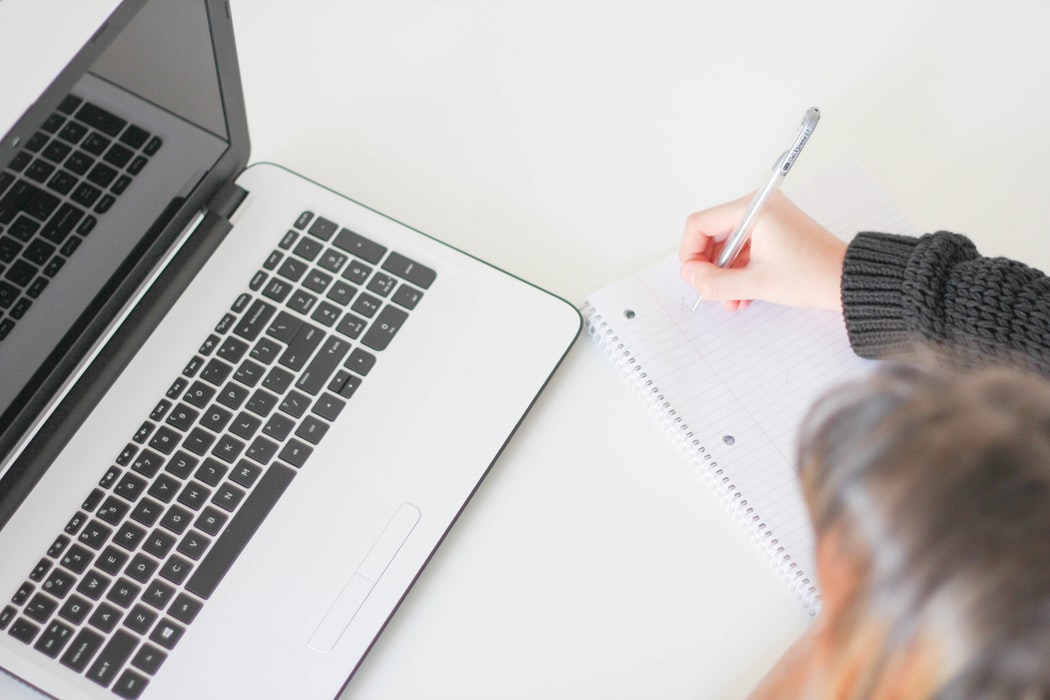
When we talk about AI, what we often refer to, is narrow AI. Narrow Artificial Intelligence refers to the AI that is hardwired to work on a specific task. We see AI being interlaced in almost every field possible, starting from industries to hospitals, from education to enterprises. In these places, all the applications of Artificial Intelligence are very specialized. Data sets involved in the learning in such AI are narrow and specific.
There is work towards creating a general AI, Artificial Intelligence which is almost like a human that can adapt and learn different varieties of tasks by learning from and creating data sets of its own to perform various specified activities at the same time. The progress in this direction has triggered the pharmacologists to work with AI in drug discovery, with the goal being trying to identify patterns concealed in large volumes of training data.
How does drug discovery work?
Drug discovery is the process in pharmacology where drugs are either designed or discovered. In earlier days, drugs were discovered from age-old remedies or simply by accident. This process wasn’t fool-proof so new methods were sought. The need to understand diseases and infections and how they’re controlled, from a physiological and molecular point of view was stressed upon. In drug discovery, a particular constituent in a compound is identified, then synthesized, tested for efficacy and finally deployed to the general population.
There are a lot of detailed processes that are carried out at every step and from the discovery to deployment with the gap of about 10 to 15 years. Drug discovery, even though an extensive field of research, is still a chance process.
A lot for experimentation is required to find a potential target for drug discovery. Once it is obtained, the identification of lead chemicals by various screening processes takes place and finally, the drug can be suitable for preclinical testing, clinical testing and then marketed to the general public. A particular aspect where AI can be useful in this entire course of action is the identification of potential targets and the extraction of lead chemicals.
Use of AI in drug discovery
- Designing an artificial chemist. Modern-day drug discovery requires the parallel comparison of various factors that influence the success of a drug on a human body. The chemical structure of the drug is almost always insufficient to account for its physiological impact. A drug often has multiple targets in a biological system and the effect of a drug is directly dependent on the genetic profile of an individual. At times, a drug has inherent compatibility issues for reasons unknown. All these factors need to be balanced while working on designing a drug. This can be handled by AI which uses machine learning algorithms to handle large data sets and classify them into various groups and work towards a common goal.
- Using RNN in collaboration with the human brain. The advantage of recurrent neural networks is that they don’t understand the chemical structures or the chemical potential of a compound. They understand the rules and patterns. With the aid of RNN, humans can specify a chemical space where RNNs generate molecules in various combinations that are drug-like by referring to the information provided beforehand. Some of these combinations result in an accurate drug target which human beings might not have thought of.
- Identification of leads. A large chemical space has a lot of places where drug targets work the best. Such places are used for the identification of lead chemicals. Using AI can quicken the hit and trial method in a smoother, more lucid manner in comparison to the human counterpart. Furthermore, it leaves the floor open to humans to work on the researching of new compounds while the AI works on the guessing game of identifying targets.
Ethical and IP Issues
For the working of AI, a large number of data sets, often called training data is required. The training data in drug discovery include sensitive information regarding the patient records and details about the disease and treatment is needed. Using this data can mean that patient privacy is compromised. Access to such data is important for personalized drugs and increased effectiveness towards disease control, but at the same time, it reveals information about the patient which can be misused by insurers or cause concern among family members.
The discovery of drugs by AI is also subject to patent issues. Considering that training data comes from rival industries, and the AI is technically not a human, it is justified that the drug discovery credit goes to the government. It cannot be patented by any patent act. However, there has been a lot of conflict on this issue whether it should remain public domain or copyrighted by some organization.
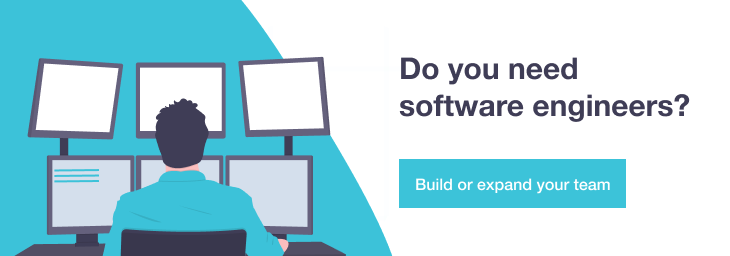
Wrapping Up – AI in Drug Discovery
Thus, AI has a lot of potential in drug discovery yet it needs to resolve certain pressing issues before it is launched in totality in the industry. AI can overcome the challenges faced by pharmaceutical companies by easy identification of targets, finding accurate molecules from a large group, suggest modifications in chemical structures and so on. Finding a way to inculcate AI in drug discovery can open floodgates of possibilities in the industry.