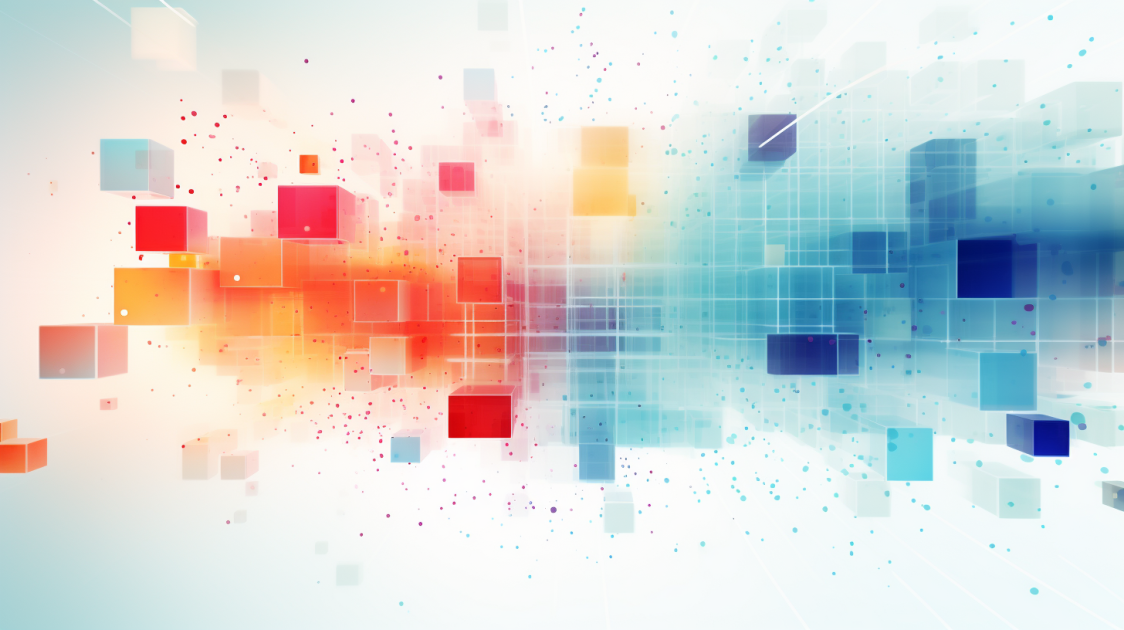
Welcome to our summary of the article “What Every CEO Should Know About Generative AI” written by McKinsey & Company. In this concise overview, we’ve distilled the key insights from the original 17-page article, saving you valuable time. Instead of spending approximately 45 minutes reading the full text, we’ve condensed the most important points into a five-minute read.
However, we encourage you to read the complete article to gain a comprehensive understanding of generative AI and its implications for CEOs. Let’s dive into the highlights and discover the transformative potential of generative AI.
Table of Contents
How Generative AI differs from AI
Generative AI is a subset of artificial intelligence that specifically focuses on creating new content or data based on patterns and existing information. It uses advanced machine learning models to generate original and realistic outputs. AI, on the other hand, is a broader field that encompasses various techniques and approaches to simulate human intelligence in machines, including generative AI.
Potential Use Cases for Generative AI
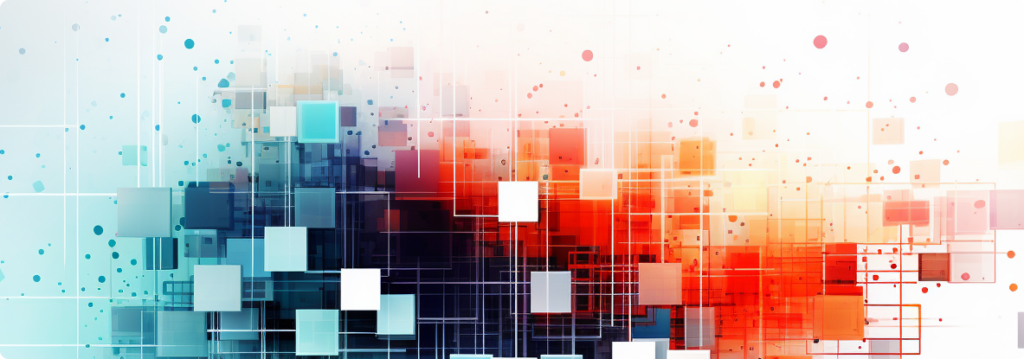
- Code Generation: Using generative AI to automate the process of writing code, improving efficiency and reducing software development time.
- Document Analysis and Synthesis: Employing generative AI to analyse and synthesise large documents, extracting relevant information and providing synthesised answers to queries, enhancing productivity for professionals.
- Customer Support and Chatbots: Implementing generative AI in customer support services to handle inquiries and provide quick responses, freeing up human representatives for more complex tasks.
- Personalised Marketing and Content Generation: Utilizing generative AI to generate personalised marketing campaigns, product descriptions, and content tailored to individual customers, improving engagement and marketing efficiency.
- Accelerating Research e.g. Drug Discovery: Applying generative AI in pharmaceutical research to analyse microscopy images and predict the relationship between drug chemistry and outcomes, accelerating the discovery process and improving decision-making.
- Virtual Experts and Knowledge Management: Developing virtual experts powered by generative AI to provide frontline workers with access to proprietary knowledge and relevant content, increasing productivity and enabling efficient knowledge sharing.
- Risk Analysis and Compliance: Leveraging generative AI to analyse and identify risks, ensure compliance with regulations, and enhance risk management processes across various industries.
- Language Translation and Natural Language Processing: Utilizing generative AI for language translation, natural language understanding, and processing textual data, facilitating communication and information extraction.
- Design and Creativity Enhancement: Using generative AI to assist in design processes, generating ideas and recommendations based on specific requirements and preferences.
- Predictive Analytics and Decision Support: Employing generative AI to analyze large datasets, identify patterns, and make predictions, enabling data-driven decision-making in areas such as forecasting and risk assessment.
Risks Associated with Generative AI
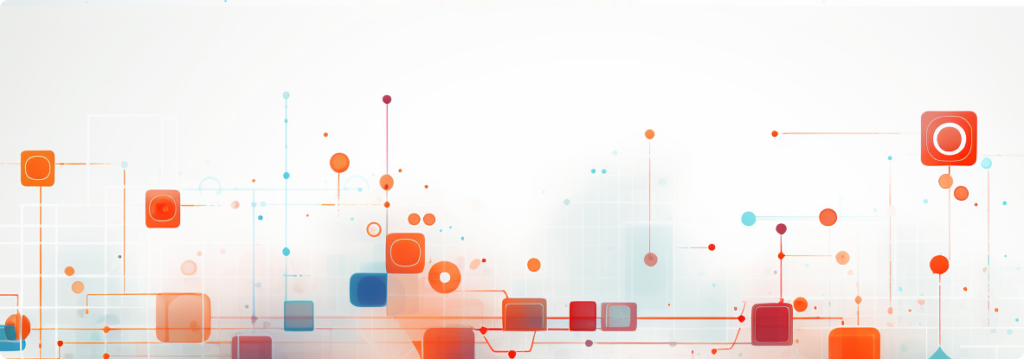
1. Vulnerabilities and Bugs in AI-Generated Code
The use of generative AI coding tools may result in code with vulnerabilities or bugs, posing risks to software quality and security.
2. Intellectual Property Violations:
There is a risk of inadvertently violating licensing and intellectual property rights when using off-the-shelf generative AI tools if the generated code is not compliant with regulations and agreements.
3. Hallucination and Inaccurate Outputs:
There is a risk of inadvertently violating licensing and intellectual property rights when using off-the-shelf generative AI tools if the generated code is not compliant with regulations and agreements.
4. Bias in Training Data:
Generative AI models trained on biased data can perpetuate and amplify existing biases, resulting in discriminatory or unfair outcomes.
5. Ethical Concerns::
Generative AI models trained on biased data can perpetuate and amplify existing biases, resulting in discriminatory or unfair outcomes.
6. Compliance and Regulatory Risks:
Organizations must navigate evolving regulations surrounding generative AI, including data protection and consumer rights, to avoid legal consequences and reputational damage.
7. Data Quality and Security:
Building and training custom generative AI models require high-quality and diverse data, necessitating privacy, security, and compliance with data protection regulations.
8. Skills and Talent Gap:
Implementing generative AI requires specialised expertise, and the lack of skilled talent can pose a risk to successful implementation and maintenance.
9. Cost and Resource Requirements:
The costs associated with generative AI vary based on the use case, development, data requirements, infrastructure, and maintenance, requiring careful resource planning.
10. User Acceptance and Trust:
Users may have concerns about AI-generated content, and building trust, transparency, and addressing resistance or scepticism is crucial for successful adoption.
Different Implementation Modes and Their Pros and Cons
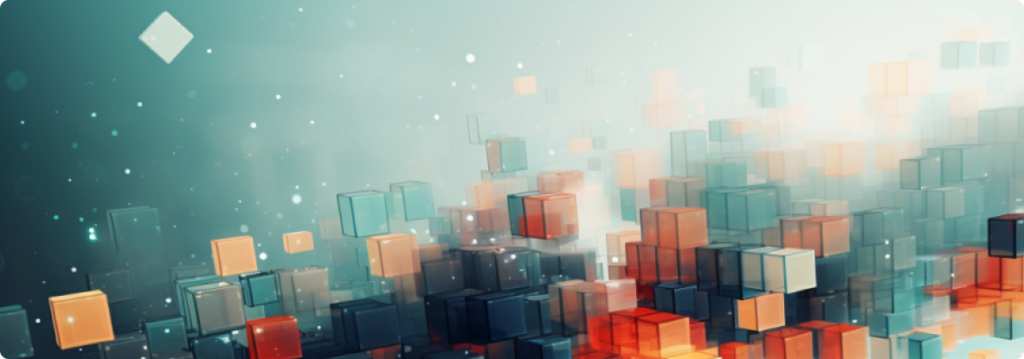
Off-the-Shelf Generative AI Tools
Pros | Cons |
---|---|
Low cost | Limited customisation and control |
quick time to market | potential vulnerabilities or bugs |
minimal development required | risk of intellectual property violations |
available expertise and support |
Building on Foundation Models via APIs or Open Models
Pros | Cons |
---|---|
Customization and adaptability | Higher investment |
integration with existing systems | development costs |
improved user experience | reliance on third-party vendors |
risk and compliance controls |
Fine-Tuning Foundation Models
Pros | Cons |
---|---|
Enhanced performance | Even higher costs |
domain-specific language alignment | specialised talent required |
improved customer satisfaction | ongoing monitoring and validation |
freeing up human resources | potential bias and inaccurate outputs |
Training Custom Models from Scratch
Pros | Cons |
---|---|
Maximum customisation and control | Substantial technical and resource challenges |
higher-performing models | highest investment |
improved efficiency | data processing and cleaning requirements |
reduced inaccuracies | rigorous testing and validation |
Areas for CEOs to Focus on When Embarking on the Generative AI Journey
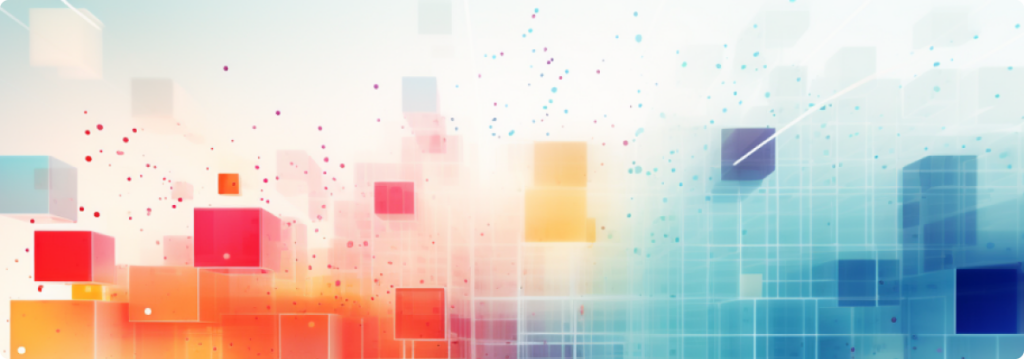
- Organisational Alignment: Convene a cross-functional leadership team to prioritise and coordinate generative AI implementation across different functions.
- Business Case Development: Identify use cases aligned with strategic goals and deliver practical benefits.
- Risk Management: Establish ethical principles, guidelines, and risk mitigation strategies to address issues like bias, privacy, security, and compliance.
- Technology Infrastructure: Ensure a modern data and tech stack with necessary resources, data systems, tools, and access to generative AI models.
- Talent Development: Identify required technical capabilities and hire or upskill employees in areas like data science, machine learning, and risk management.
- Partnership and Ecosystem Approach: Build alliances with generative AI vendors, model providers, and infrastructure providers to accelerate execution.
- Culture of Innovation: Foster a culture of self-driven research and experimentation, and provide guidelines and training on generative AI tools.
- Start Small, Learn, and Scale: Begin with small proofs of concept, focus on early wins, and gradually scale generative AI implementation.
- Continuous Learning and Adaptation: Stay updated on generative AI technology, regulations, and best practices, and continuously adapt the organisation’s approach.
In conclusion, generative AI offers CEOs transformative opportunities but also carries risks. By prioritising alignment, developing strong business cases, and addressing ethical considerations, CEOs can effectively leverage generative AI to drive innovation and stay competitive in the ever-changing business landscape.