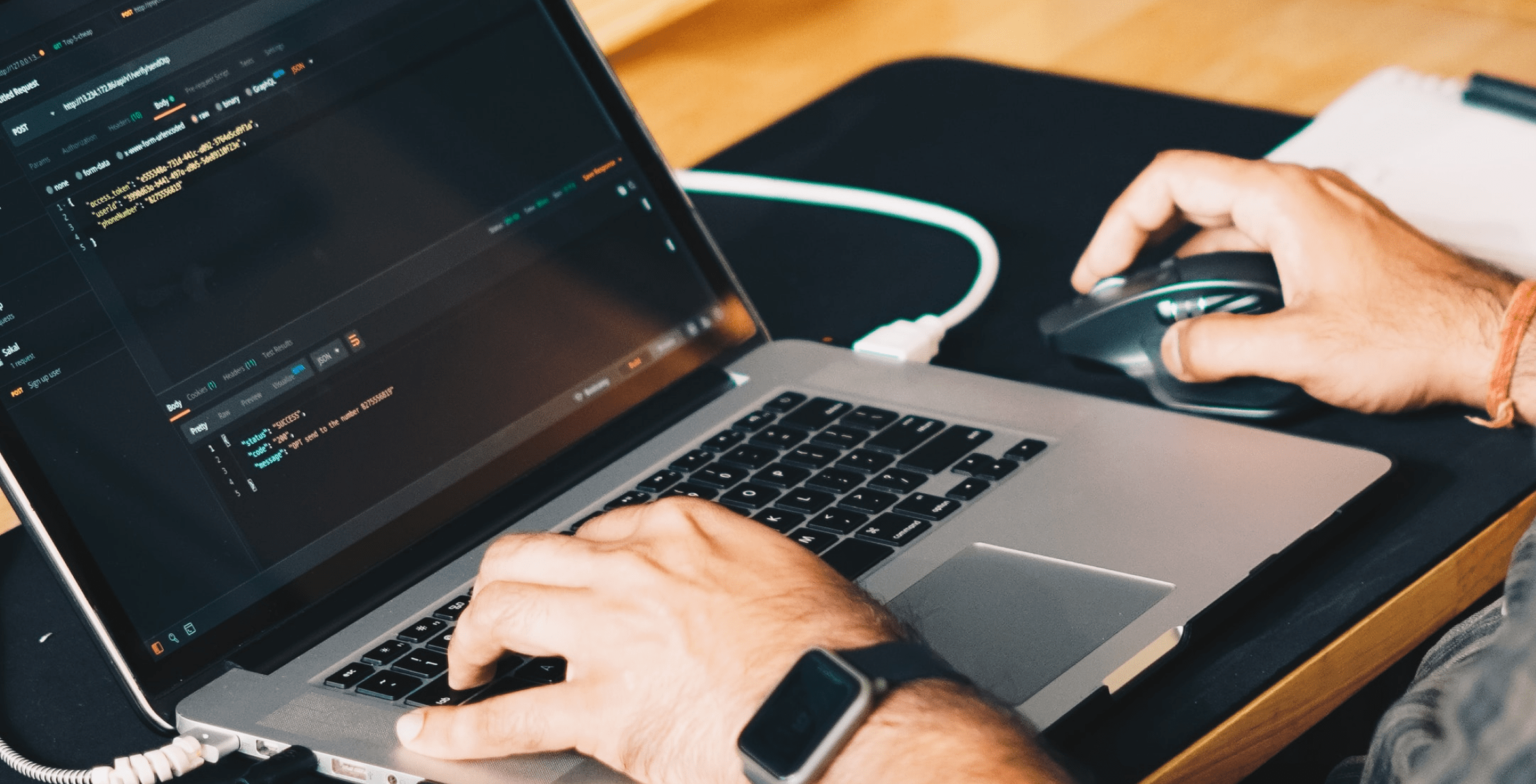
Businesses all over the world are going through digital transformation and becoming more data-driven to gain a competitive advantage. It requires adopting the newest technologies and solutions. AI importance grows in basically all economic sectors. Is it a good idea to use machine learning for data science?
Your organization is probably collecting terabytes of data every minute. You cannot process it effectively without proper technologies and tools. The way to analyze it efficiently is by adopting AI machine learning for data science. Before you decide to transform your business entirely, you need to learn more about data science, machine learning, and how they can be used. Take the moment to consider how many small, medium-sized and large companies invest in some sort of AI-based tools for business. You will soon realize that you are surrounded by data-driven organizations and to keep up, you need to use advanced solutions as well.
Data Science and Machine Learning — differences
Although data science and machine learning are related, they are not the same thing. Data science is an umbrella term for the processes and methods that allow data scientists to transform data into useful insights. It is a broad field in between information technology and science. Techniques used in data science can be leveraged for many purposes (business or research). The basis of the data scientists’ work is always information in digital form.
Machine learning is a subset of Artificial Intelligence, and it is a collection of techniques and algorithms that are indispensable for creating software that possesses the ability to learn. Nowadays, ML gains popularity in the world of business (in various industries) along with advanced, AI-based analytics.
Here are some most important things you should know about data science and machine learning:
- Data science is about analyzing data and producing insights, whereas machine learning’s goal is to make machines capable of learning on their own from the numerous data sets provided by data scientists.
- Training machine learning algorithms with structured data is easier than with unstructured one. In data science, all types of data are used.
- Data science and machine learning require different skills from experts.
Machine Learning for Data Science — how is ML used in data science?
Machine learning and artificial intelligence can be used in data science. It allows for the automation of data analysis processes and makes it possible to produce predictions in real-time without human supervision. Properly trained data models may handle it entirely on their own. ML engineers must provide relevant data, define the specific features of the particular data model, and then train it.
Machine learning in the data science lifecycle consists of the following steps:
- Collecting data — you cannot start using ML without providing the data sets necessary to train your ML models. You need to gather relevant information first.
- Preparing data — gained data must be made ready for analysis. Data cleaning includes eliminating incomplete and irrelevant pieces of information or fixing them (if possible). All data used for ML must be standardized (transformed into a unified format).
- Training ML model — at this stage, the gathered information is used to train a data model. The output will probably not be “as expected” at the very beginning of the training, but the learning continues and there is sometimes a need for multiple adjustments.
- Testing ML model — after training your data model, you need to assess its performance. The model has to be fed with fresh data that has never been used. In this step, you can observe how your machine learning model works with completely new information. This allows you to find out how your model performs in real-life applications.
- Deploying the data model — the final step. Now, the data model can be used in the way it was meant to be to answer various users’ questions.
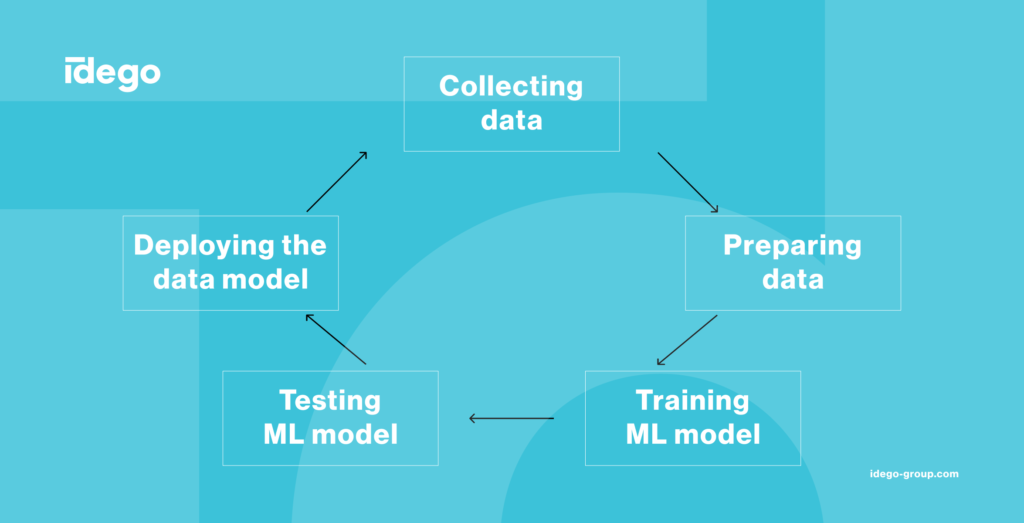
There are many machine learning algorithms used in data science, for example, linear regression, logistic regression, decision trees, naive bayes, K-Means clustering, and others. The choice of algorithm depends on the ML project.
Read also: Extracting data from documents using latest Machine Learning techniques
Statistics for data science and machine learning
According to the Statista reports, the adoption of data science and ML technologies in research and development is the fastest growing trend among enterprise departments. Around 71% of respondents from R&D said that they applied data science and ML-based solutions in their work in 2019. Still, ML and data science help solve problems in other departments too.
Companies use machine learning in many ways. In recent years, ML algorithms were used to predict the mortality of the patients infected with the COVID-19. The accuracy of the ML predictions was 92%. Machine learning proves to be a technology that is capable of providing reliable insights for any type of organization. Professionals all around the world started to realize that already some years ago. The NewVantage Partners 2020 Survey informs that 91.5% of surveyed US firms report ongoing investment in AI.
How are data science and ML used in business? According to the data from Statista from 2019, 33% of surveyed IT leaders planned to use ML for business analytics, 25% wanted to leverage ML for cybersecurity improvements, sales, and marketing purposes (16%), and customer services (10%). How exactly are data science and machine learning useful for business purposes?
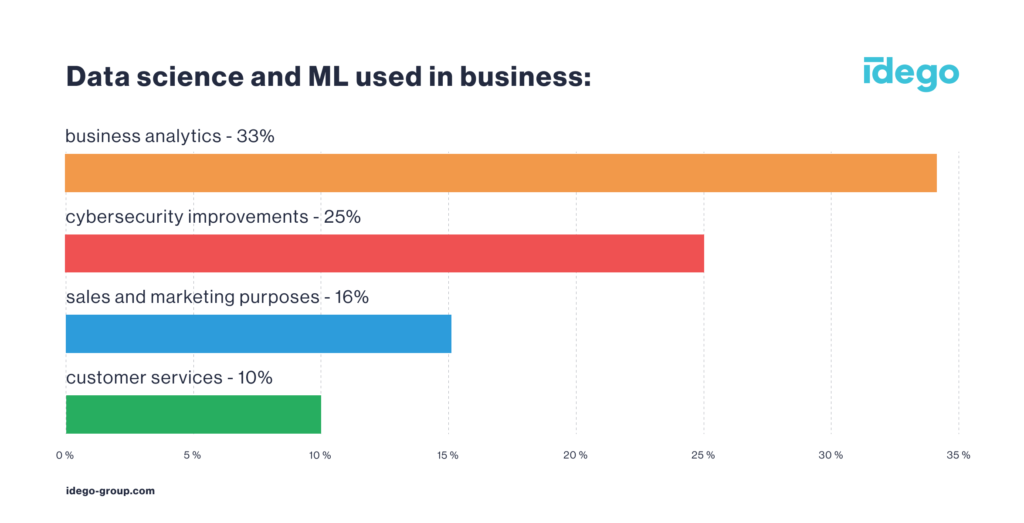
Which projects require data science with machine learning?
Machine learning and data science have multiple applications in almost every sector of industry and science. They have been used in so many popular apps and tools we use every day to make our lives easier (like for example Alexa). You can adopt ML-based solutions in your business to:
- Detect fraud — fraud detection is a very important ML used for financial institutions and banks. Machine learning models can be trained to analyze real-life transactions compared to the transaction patterns, recognize and flag any transaction that appears suspicious.
- Prevent cybercrime — similarly, data models can be taught to spot abnormal activity in a company’s systems. The most advanced cybersecurity software relies on artificial intelligence and machine learning.
- Recognize speech — machine learning allows us to teach machines and applications to understand different human languages and accents. The sounds are being converted into words, so the application could analyze them and come with the relevant response for the user. Speech recognition has been used by various virtual assistants for many years already.
- Recommend products, services, or solutions — online recommendation engines are very popular, especially in e-commerce. They are powered by ML algorithms and capable of suggesting products to buy to the e-commerce app users. How does it work? Machine learning models can analyze the customer’s previous behavior (shopping choices, viewed items, historical purchases) and any other information about the client’s potential preferences. Based on that recommendation, the system selects the items that the customer can be interested in and shows them to the buyer.
Data science and machine learning have enormous potential in any industry. Would you like to adopt advanced, ML-based solutions in your company? We can help you with that. Contact us and tell us about your vision or ask us about our best ideas for your business.